文章来源:Jianxiao Wang, Liudong Chen, Zhenfei Tan, Ershun Du, Nian Liu, Jing Ma, Mingyang Sun, Canbing Li, Jie Song, Xi Lu, Chin-Woo Tan, Guannan He. (2023). Inherent spatiotemporal uncertainty of renewable power in China. Nature Communications: 14, 5379. https://doi.org/10.1038/s41467-023-40670-7
整理人:徐嘉苗,2021级本科生
整理时间:2024年6月5日
Abstract: Solar and wind resources are vital for the sustainable energy transition. Although renewable potentials have been widely assessed in existing literature, few studies have examined the statistical characteristics of the inherent renewable uncertainties arising from natural randomness, which is inevitable in stochastic-aware research and applications. Here we develop a rule-of-thumb statistical learning model for wind and solar power prediction and generate a year-long dataset of hourly prediction errors of 30 provinces in China. We reveal diversified spatiotemporal distribution patterns of prediction errors, indicating that over 60% of wind prediction errors and 50% of solar prediction errors arise from scenarios with high utilization rates. The first-order difference and peak ratio of generation series are two primary indicators explaining the uncertainty distribution. Additionally, we analyze the seasonal distributions of the provincial prediction errors that reveal a consistent law in China. Finally, policies including incentive improvements and interprovincial scheduling are suggested.
摘要:太阳能和风能资源对于可持续能源转型至关重要。尽管现有文献中广泛评估了可再生能源潜力,但很少有研究考察源于自然随机性的内在可再生不确定性所固有的统计特性,而这种随机性在涉及随机性的研究与应用中是不可避免的。本文开发了一种用于风能和太阳能发电预测的经验法则统计学习模型,并生成了中国30个省份长达一年的每小时预测误差数据集。我们揭示了预测误差的多样化时空分布模式,表明超过60%的风能预测误差和50%的太阳能预测误差源自高利用率场景。发电序列的一阶差分和峰值比是解释不确定性分布的两个主要指标。此外,我们分析了省级预测误差的季节分布,揭示了中国境内的一致规律。最后,本文建议了包括激励机制改进和省际调度在内的政策建议。
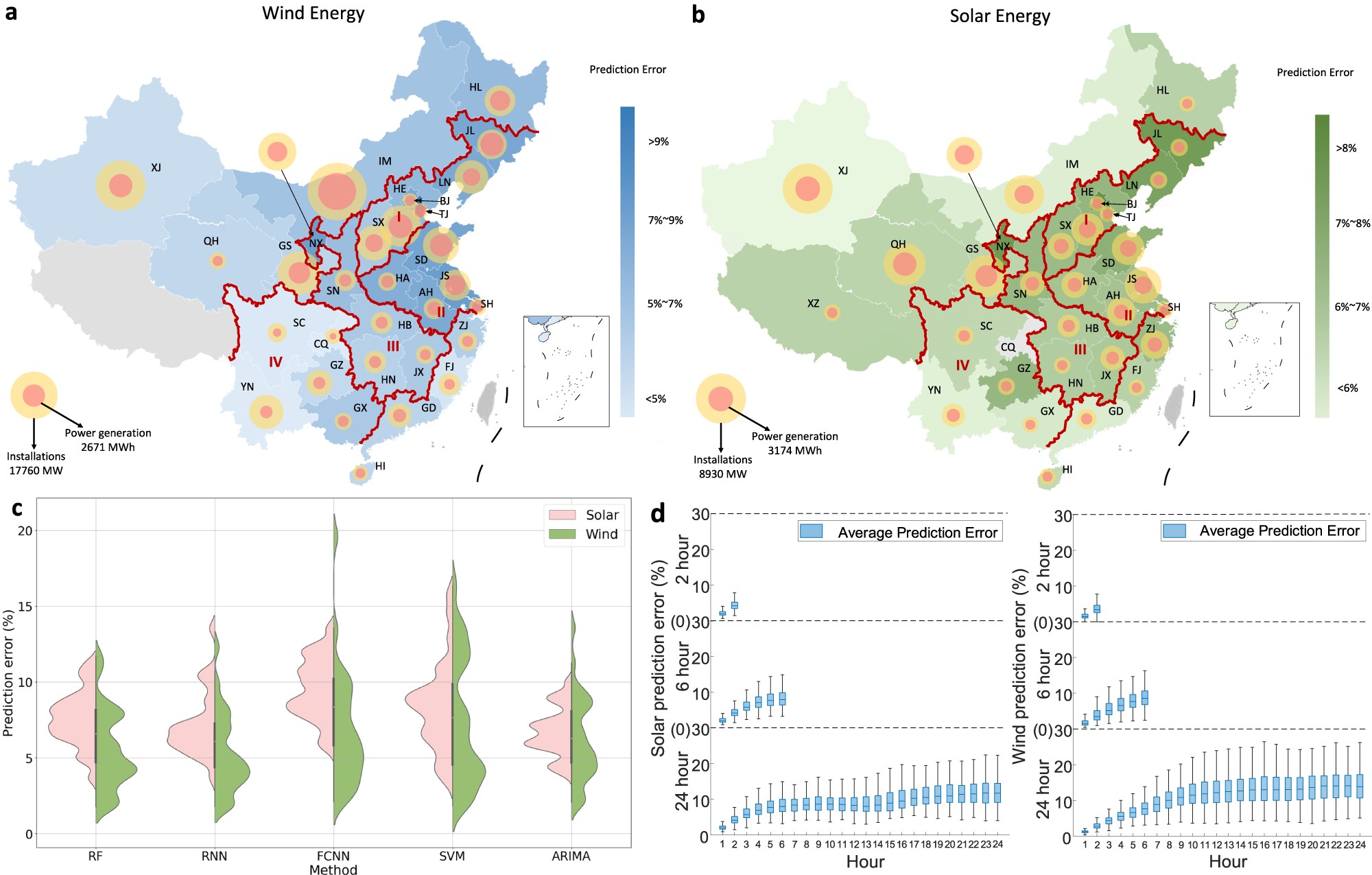
图 风能和太阳能功率预测误差的空间分布以及不同方法和时间尺度的影响
注:a风电预测误差空间分布;b光伏预测误差空间分布;c不同预测算法的误差分析;d不同预测时长的误差分析
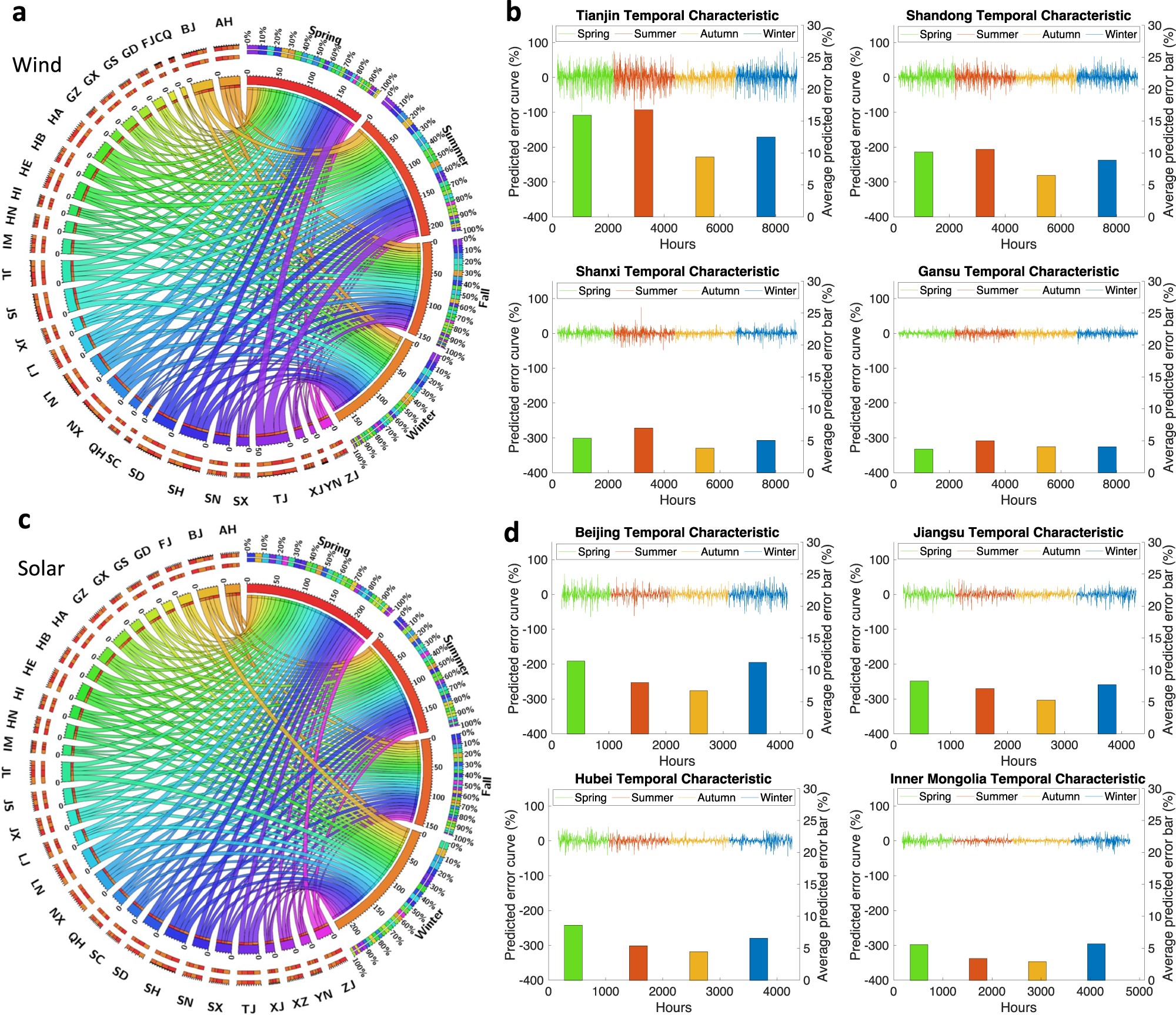
图 风能与太阳能预测误差的时间序列分析
注:a不同季节各省风电预测误差;b典型地区风电预测误差季节性规律;c不同季节各省光伏预测误差;d典型地区光伏预测误差季节性规律
原文链接:https://www.nature.com/articles/s41467-023-40670-7
节选转引:https://mp.weixin.qq.com/s/dxxDcuETG3b959sLakhVgw