文章信息:Plésiat, É., Dunn, R.J.H., Donat, M.G. & Kadow, C. (2024). Artificial intelligence reveals past climate extremes by reconstructing historical records. Nature Communications, 15, 9191. https://doi.org/10.1038/s41467-024-53464-2
整理人:闫甲祺,2024级硕士研究生
整理时间:2024年12月10日
Abstract: The understanding of recent climate extremes and the characterization of climate risk require examining these extremes within a historical context. However, the existing datasets of observed extremes generally exhibit spatial gaps and inaccuracies due to inadequate spatial extrapolation. This problem arises from traditional statistical methods used to account for the lack of measurements, particularly prevalent before the mid-20th century. In this work, we use artificial intelligence to reconstruct observations of European climate extremes (warm and cold days and nights) by leveraging Earth system model data from CMIP6 through transfer learning. Our method surpasses conventional statistical techniques and diffusion models, showcasing its ability to reconstruct past extreme events and reveal spatial trends across an extensive time span (1901-2018) that is not covered by most reanalysis datasets. Providing our dataset to the climate community will improve the characterization of climate extremes, resulting in better risk management and policies.
摘要:对近期极端气候事件的理解和气候风险的特征描述需要在历史背景下研究这些极端事件。然而,由于空间外推不足,现有的观测极值数据集通常表现出空间差距和不准确性。这个问题源于用于解释缺乏测量的传统统计方法,特别是在 20 世纪中叶之前普遍存在。在这项工作中,我们通过迁移学习利用 CMIP6 的地球系统模型数据,利用人工智能重建欧洲极端气候(温暖和寒冷的白天和夜晚)的观测结果。我们的方法超越了传统的统计技术和扩散模型,展示了其重建过去极端事件并揭示大多数再分析数据集未涵盖的广泛时间跨度(1901-2018)的空间趋势的能力。向气候界提供我们的数据集将改善极端气候的特征,从而实现更好的风险管理和政策。
1.研究背景
2.研究意义
3.研究方法
Climate Reconstruction AI (CRAI):基于U-Net和部分卷积层的深度学习技术,用于重建气候极端指数。
数据训练与评估:使用CMIP6档案中的地球系统模型(ESMs)的历史模拟数据训练AI模型,并用ERA5、HadEX-CAM和CMIP6模型的数据进行评估。
比较评估:将CRAI的结果与其他插值方法(IDW、Kriging和扩散模型)进行比较,使用均方根误差(RMSE)、Spearman秩相关系数(SROCC)、Wasserstein距离(WD)和决定系数(R²)等指标。
4.主要结论
CRAI性能优越:CRAI在所有指标和数据集上的重建性能均优于其他方法,尤其是在处理大量缺失数据时。
空间趋势分析:CRAI重建的数据集显示了与HadEX3不同的空间趋势,提供了更详细的区域变化视图。
早期极端天气事件:CRAI模型能够更清晰地揭示早期极端事件,如1911年的热浪和1929年的寒潮,与历史记录和其他代理指标一致。
5.研究展望
全球应用潜力:研究展示了将这种方法应用于全球尺度或其他数据稀缺地区的潜力。
数据集成:将更多相关数据集作为CRAI模型的输入,可能通过利用稀疏数据区域的相关信息进一步改善极端指数的重建。
神经网络下尺度化:使用神经网络下尺度化有望揭示更复杂的历史气候模式。
每日数据训练:用每日原始测量数据训练CRAI模型,以投影月度ETCCDI指数,可能通过利用更多信息提高准确性。
6.研究主要图表:
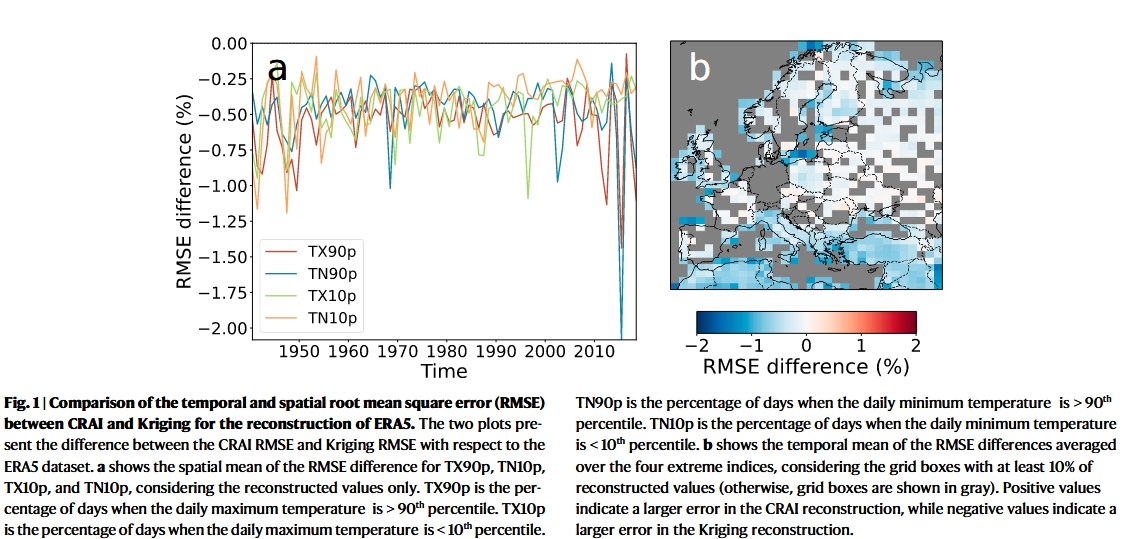
01 CRAI 与 Kriging 重建 ERA5 时空均方根误差 (RMSE) 的比较
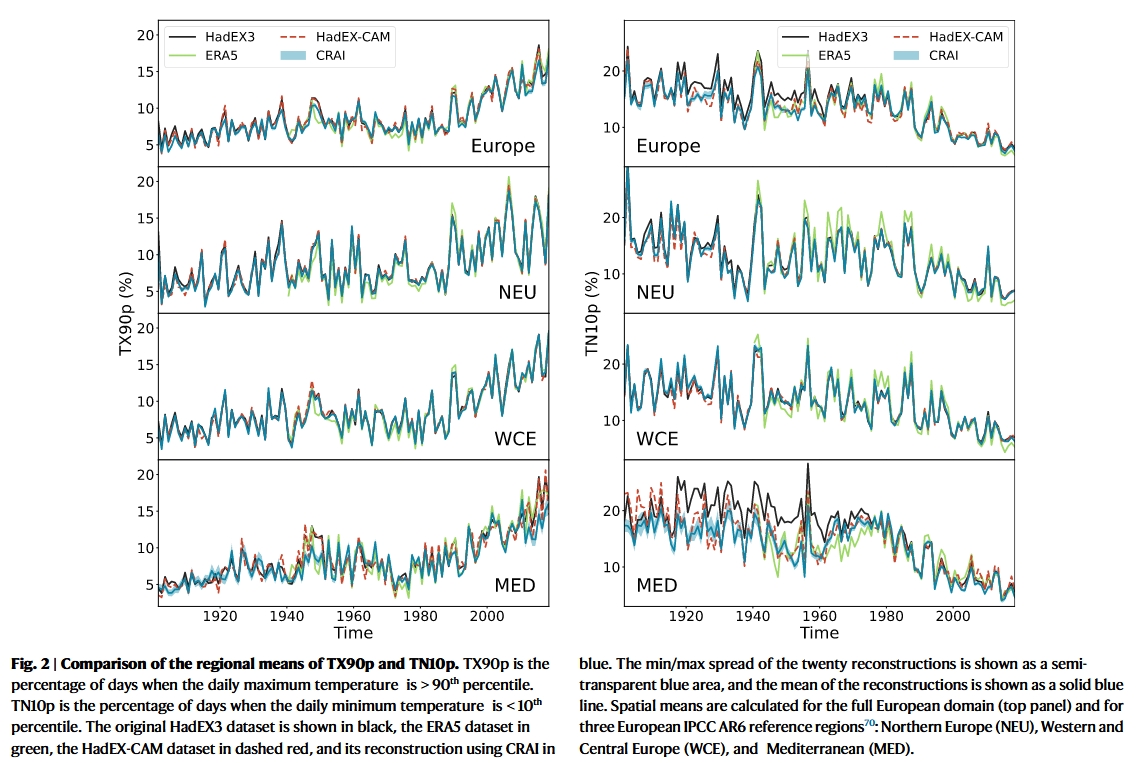
02 TX90p 和 TN10p 的区域平均值比较
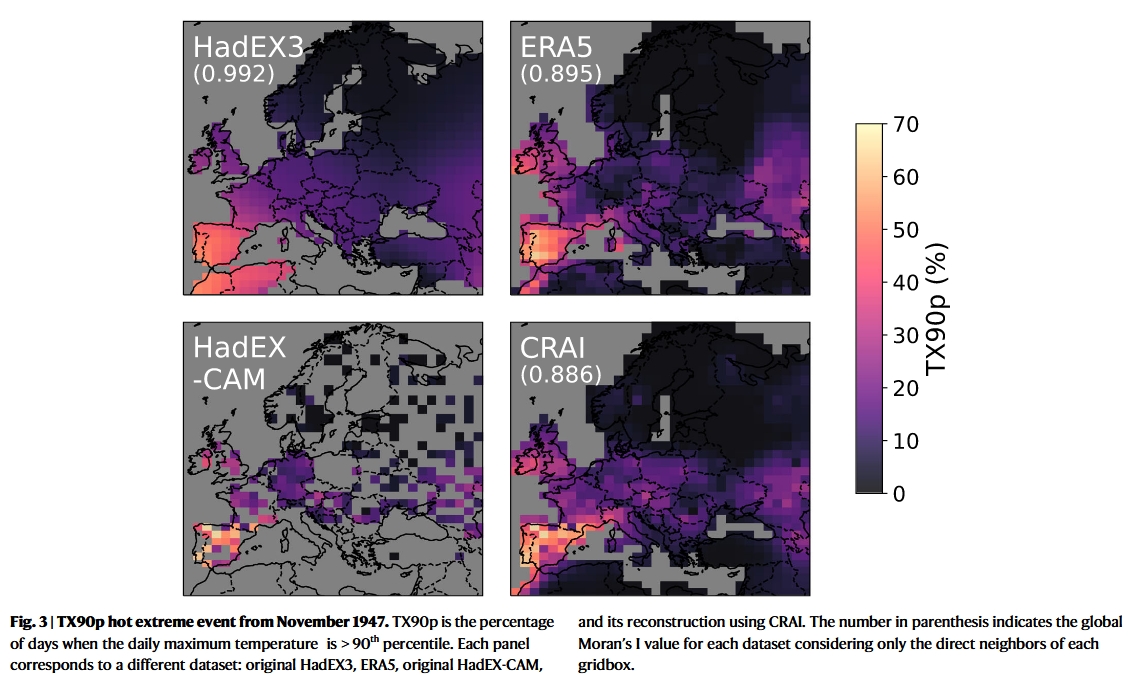
03 1947 年 11 月以来的 TX90p 高温极端事件
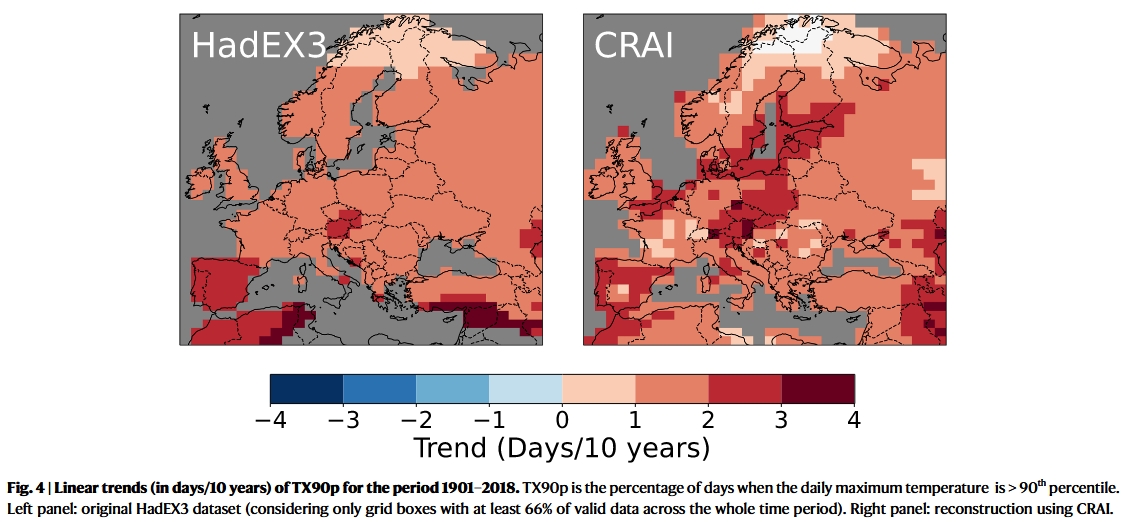
04 1901-2018 年期间 TX90p 的线性趋势(以天每10 年为单位)
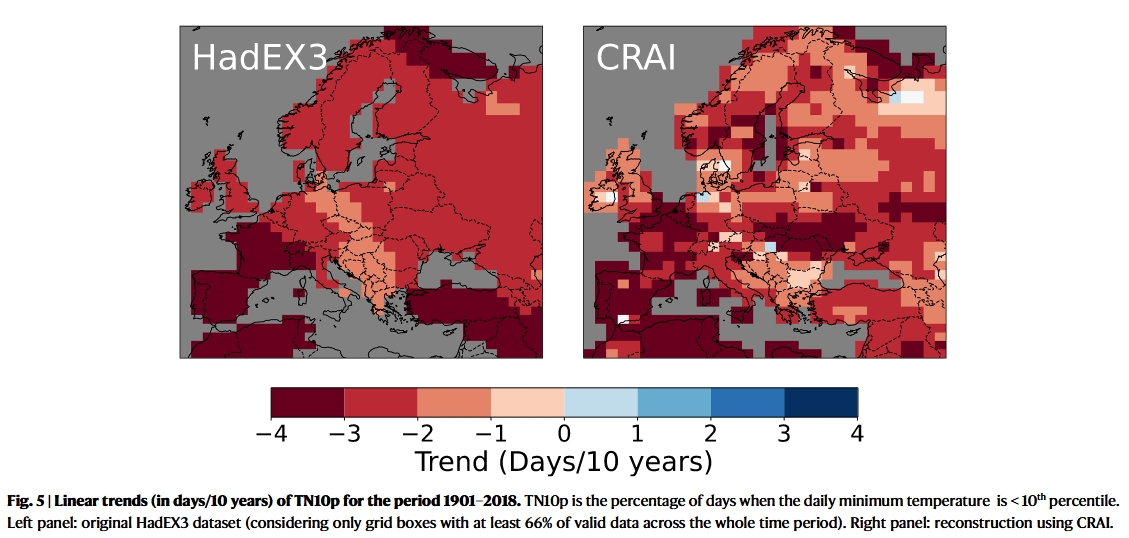
05 1901-2018 年期间 TN10p 的线性趋势(以天每10 年为单位)
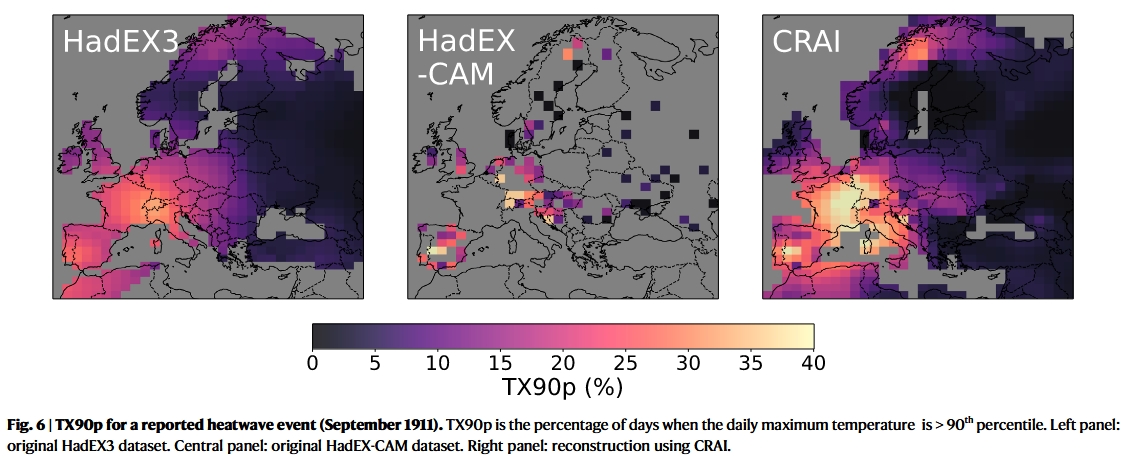
06 TX90p 用于报告的热浪事件(1911 年 9 月)
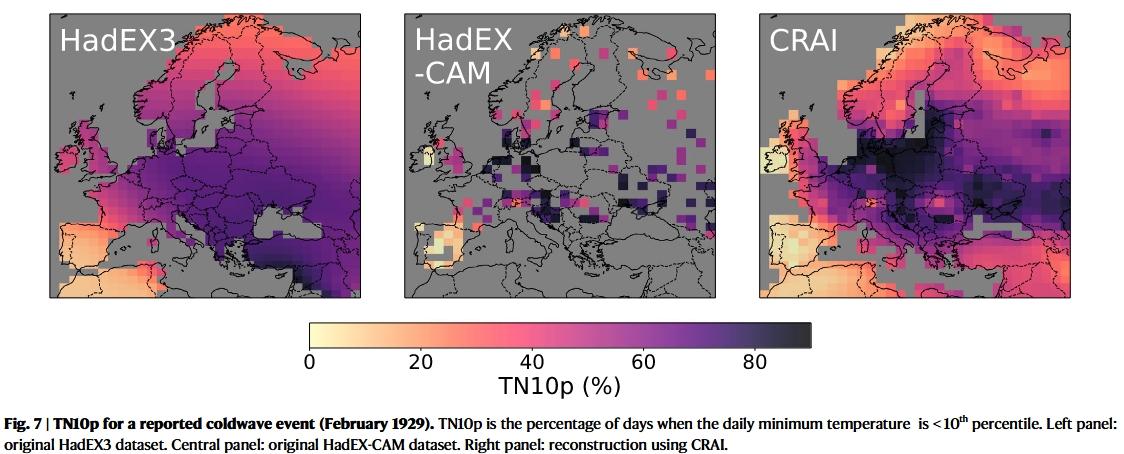
07 TN10p 表示已报告的寒潮事件(1929 年 2 月)
原文链接:https://www.nature.com/articles/s41467-024-53464-2
原文转引:https://mp.weixin.qq.com/s/p33OV-mZ_J3bhhPoaswT1Q